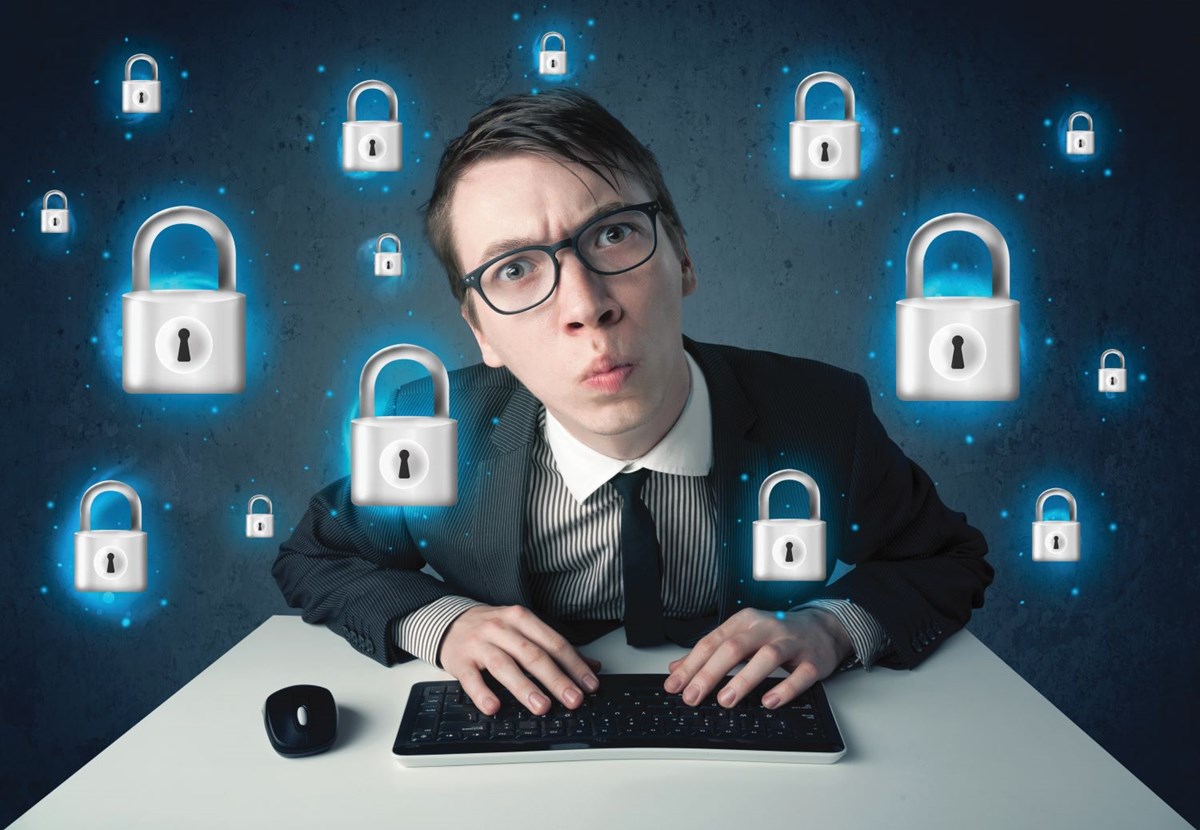
If they haven’t already, cybercriminals wielding machine learning algorithms will be coming for your financial and health information, your self-driving car and your online reputation—even as other machine learning programs try to protect them, says Computer Science Asst. Prof. Yimin (Ian) Chen, who does research on using machine learning to improve cybersecurity.
“They are getting stronger on the dark side, too,” he says. “As machine learning evolves, they try to bypass our defenses.”
Chen says one powerful tactic is “adversarial example attacks,” in which an attack algorithm figures out the smallest possible change to an image that will lead a machine learning network to misclassify it. A typical scenario would be to tamper with a self- driving car so its imaging software misreads a stop sign as a 25-mph speed limit sign; soon, other autonomous vehicles on the same manufacturer’s machine learning network could start running stop signs.
Deepfakes are another widespread example of malicious machine learning programs making realistic images, videos and audio from existing samples, Chen says, such as the realistic-looking but faked nude photos of celebrities that have flooded social media sites recently.
Digital networks will always have some vulnerabilities because they are open systems by design, Chen says. That’s because AI virtual assistants like Alexa and Siri, social media apps and health care portals all need some of your private information to be useful.
But it’s not all bad news. “Machine learning can amplify attacks, but it can help protect against them, too,” Chen says. His own research involves using AI to better detect and block spam accounts in online marketplaces.
Going forward, Chen would like to explore how distributed computing, such as block-chain, can bolster digital privacy and security. “Some people want to walk away from centralized computing,” he says. “They don’t want Big Brothers that gather all this data, as they have little faith that the data will not be misused.”
But government, academics and private companies are all working on the problem, he says.
In UML’s Miner School of Computer & Information Sciences, Prof. Xinwen Fu works on making wireless networks more secure, especially the “Internet of Things” (appliances and other household items with network connectivity), and Asst. Prof. Sashank Narain studies ways to prevent criminals from exploiting smartphone sensors to steal data and stalk people.
Thanks to them and other faculty who study machine learning, UML is a leader in cybersecurity education—not only within computer science, but also in partnership with the School of Criminology and Justice Studies, which offers a master’s degree in security studies with a cybersecurity concentration.
UML was the first public university in the Northeast to open a Cyber Range, which features 20 networked computers that can safely launch cyberattacks against each other. The Cyber Security Club, coached by Chen last year, hosted the 2023 Northeast Collegiate Cyber Defense Competition, which Narain organized.
Chen thinks academics and students will develop new cybersecurity tools, especially apps that use machine learning to fortify against the biggest vulnerability in all cyber-security systems: human error. One example would be an app that could quickly preview where a “click here” link will actually take you, he says.
“Machine learning might do most of the work of defending against attacks, and then it might make more personalized recommendations to individual users,” he says. “If everyone practices cybersecurity, then we create a very high bar for attackers.”—KW